Autor
David Fehrenbach
David is Managing Director of preML and writes about technology and business-related topics in computer vision and machine learning.
While usually working with precasted concrete elements, we joined forces with Dr. Ravi A. Patel and Dr.-Ing. Frank Dehn from the “Institute for building materials and concrete construction (IMB)” at KIT to apply our skills to existing buildings, specifically bridges.
The conference paper with the title “Convolution neural network-based machine learning approach for visual inspection of concrete structures” was published this month (January 2022) in “Proceedings of the 1st Conference of the European Association on Quality Control of Bridges and Structures”.
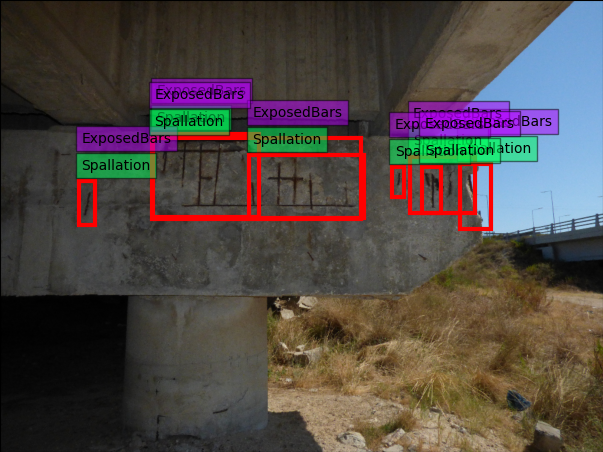
Here some insights from the work as a summary:
Problem: Manual visual inspection of concrete while being important for both quality control and maintenance is a expensive, time consuming and labour-intensive task.
Possible Use Case: In this context, machine learning aided robotic systems can help to automate visual inspection.
Purpose of the paper: There is already research in machine learning based solutions for visual inspection focuses on detection of cracks. Nevertheless, intelligent visual inspection systems for concrete structures should not only be able to detect damage but classify them and provide insights into the origin of damage by predicting one or more additional labels for each defect.
Design: To enable the development of such intelligent systems we first provide an outline of a conceptual continuous learning framework where a web hosted annotation tool act as intelligence gathering interface. The preliminary bounding box-based convolution network for damage identification and classification within this framework acts as an assistant through which active learning will become more robust in the future. We further develop and train such a network on the open access database CODEBRIM (for details see Figure 2). The main architectural contribution is the novel multi-label classification head, which not only classifies the defects but also assign it one or multiple additional attributes.
Result: We find that the developed network is helpful for assisting with the annotation task. Further it might be interesting, that our developed network has sufficient accuracy reached a mean multi label accuracy of 92.7% (for details see Table 1).
If you are interested in reading the full text, feel free to contact us via contact@preml.io anytime and we will send it to you.
Big thank you to Dr. Patel & Dr.-Ing. Dehn from IMB as well, we will continue ti work together in this and other topics merging artificial intelligence and concrete and will keep you updated on the proceedings!
Attachements:
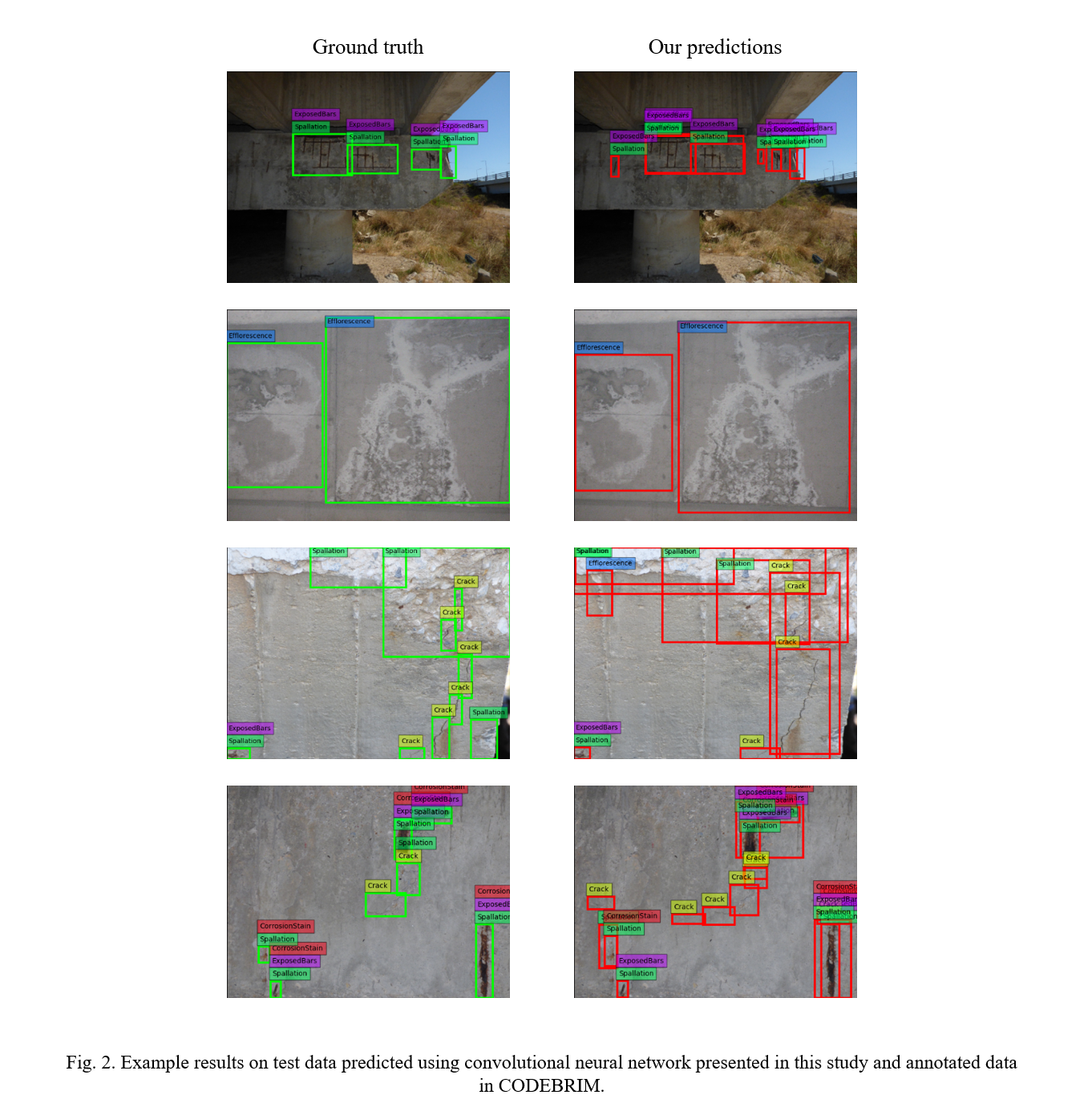
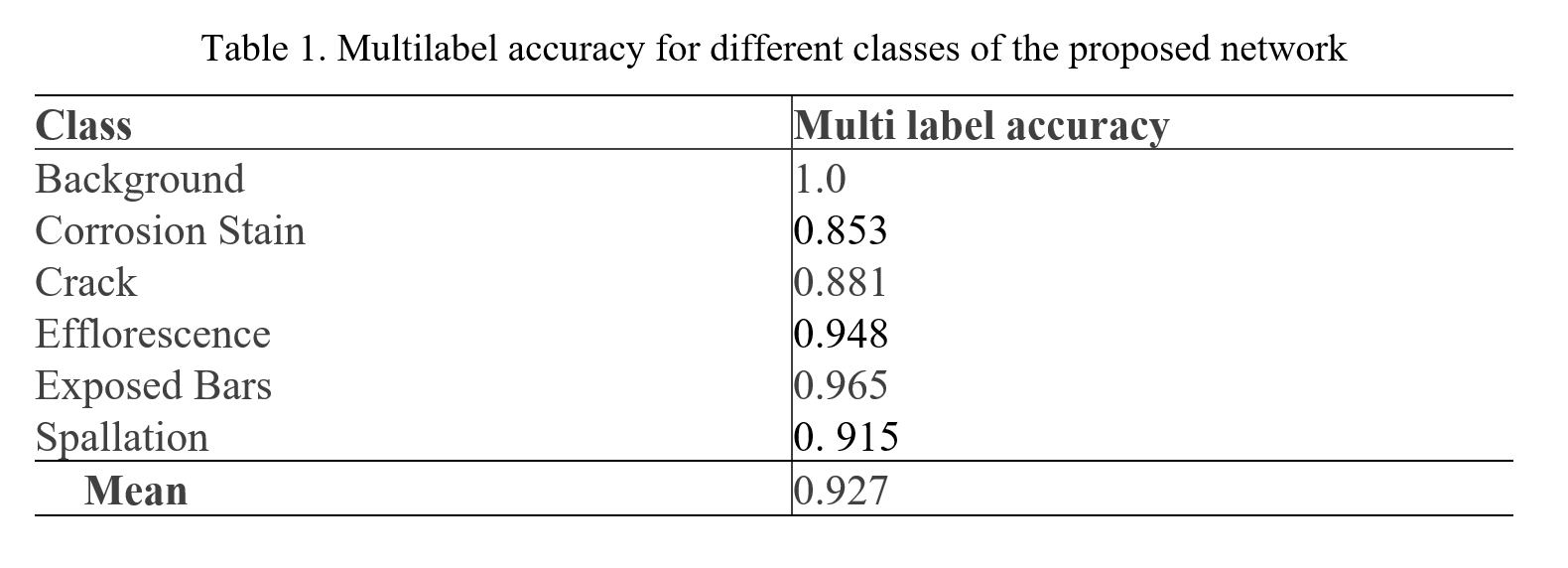
Image source: Patel R.A., Steinmann L., Fehrenbach J., Fehrenbach D., Dehn F. (2022) Convolution Neural Network-Based Machine Learning Approach for Visual Inspection of Concrete Structures. In: Pellegrino C., Faleschini F., Zanini M.A., Matos J.C., Casas J.R., Strauss A. (eds) Proceedings of the 1st Conference of the European Association on Quality Control of Bridges and Structures. EUROSTRUCT 2021. Lecture Notes in Civil Engineering, vol 200. Springer, Cham. https://doi.org/10.1007/978-3-030-91877-4_80
Autor
David Fehrenbach
David is Managing Director of preML and writes about technology and business-related topics in computer vision and machine learning.