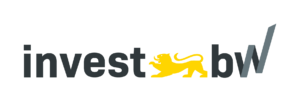
Synthesis of AI training data for visual quality inspection with object properties from CAD data
This research project aims to address the limiting factor of collecting sufficient image data for visual quality inspection with artificial intelligence by developing an approach that automatically converts 3D CAD data into an image dataset with defects and annotations, utilizing photorealistic synthetic data and generative textures.
Timeline: 02/2023 – 06/2024
Collecting sufficient image data is one of the limiting factors in visual quality inspection with artificial intelligence (AI). For each defect type, the manufacturing company must provide at least 30 sample images so that an inspection can be performed with high accuracy. Especially in view of the ongoing individualization of the production with many thousands of product variants, it is often uneconomical to collect enough examples to cover all possible eventualities. One way to counteract this problem is learning from photorealistic synthetic data, which has been increasingly investigated in AI research in recent years. This involves using computers to automatically generate large sets of artificial images based on only a small amount of input data.
However, current approaches to using synthetic data involve a lot of manual work (especially graphics design) to adapt to individual use cases and are often limited in their variability to the position and orientation of objects, as well as the type of background. For application in visual quality inspection, this approach needs to be extended to work at the sub-object and material level as well, making the technology applicable to new products in an automated way.
.
This research project follows the approach to automatically convert existing 3D CAD data into a large image dataset with defects and associated annotations. For this purpose, the appropriate material is automatically selected from a library of materials based on the material information in the CAD model. Random defects can be created by using generative textures.
The exploitation of the research results is planned in the form of a software module in the internal software architecture of preML GmbH, as well as a new software product to be developed with a user interface, which is aimed directly at users from industrial companies. The use of CAD data for the creation of a synthetic image dataset enables companies with high product variations in particular to use AI-based quality inspection systems. This enables a more efficient production, which reduces the costs for the companies, as well as an important contribution to the conservation of resources and thus to the important CO2 reduction through the early defect detection enabled by this.
The project was a sole project by preML GmbH. The research result further support the development of our CVC Inspect Software, our core product for solving visual quality inspection tasks.
#syntheticData #computerVision #machineLearning #visualQualityControl